There’s a lot of loose talk these days about Artificial Intelligence. The machines are on the verge of taking over. Driverless cars are speeding around the corner. Deep learning systems can not only beat the best players at Go or whatever, but invent entirely new kinds of strategy that mere humans can’t even comprehend. Algorithmic trading convulses the financial markets. Algorithmic videographers fill YouTube with child torture porn. Russian bots stampede across the social internet and Cambridge Analytica’s magical mind control powers will make you vote for things you otherwise wouldn’t dream of. Facebook knows where you live, your favourite food, how often you talk to your mother, those classic books you pretend to have read but haven’t, how much you hate your job, who you fancy, your history of teenage shoplifting, that unusual position you like to have sex in. Amazon have already packaged up the rope you’re soon going to order to hang yourself in the face of this all-consuming technocapitalist dystopia. They know you’re ready to do it, you just haven’t — quite — realised it yet.
Here, have a line of Charlie.1
This is not, exactly, all bollocks. There are plenty of things to be concerned about in the disposition and applications of machine learning and data mining and automation. But also, most of those things are really just tinselly avatars of the same old social problems that run through our societies like seaside resort names through pink minty rock. Just the latest electro remixes of such hoary trad standards as inequality and division and greed and exploitation. Sing out, Louise!
And meanwhile, we’re supposed to quake in fear of advertisers drip feeding us with perfectly tailored streams of opium-laced gripe water when what that actually means is this:
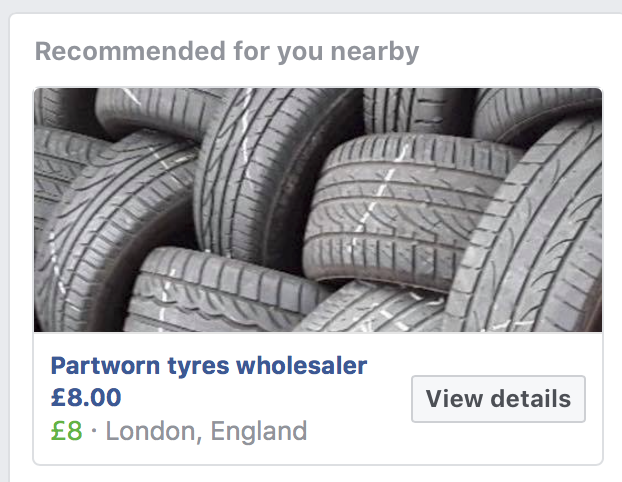
Targeted advertising is terrible. Recommendation engines are terrible. Amazon turns over billions of dollars a year and the best it can fucking do is suggest you buy slightly different editions of books it has sold you already.2
While it’s true that there have been all sorts of technical breakthroughs in AI-related endeavours in recent years, in some important ways the state of the field is not all that different from what it’s been since the early days of Marvin Minsky, Seymour Papert et al. Perhaps the most consistent feature of AI throughout its history has been how incredibly bad we are at understanding what is easy and what is hard. We have been tuned by millions of years of evolution to do a lot of incredibly difficult things without a second thought, while finding others — which might be much easier, harder, functionally identical, whatever — fucking impossible. Those natural abilities and disabilities are highly contingent on what turned out to be handy for survival and procreation on the prehistoric veldt, and bear essentially no relation to what a machine can or can’t do well.
One of those things — arguably the defining characteristic of human intelligence3 — is the ability to jump from the specific to the general. This is so profoundly built into how we think that we are almost incapable of considering any problem in isolation. The exact opposite is true for machines. Machines generalise very badly, but that failure is so alien to our way of thinking that we never properly understand it, even when we actively try.
Every time we see a machine solve some specific really difficult problem — which happens all the time because machines are getting really powerful and we’re getting pretty good at posing specific really difficult problems in ways that are susceptible to that power — we can’t help but assume it generalises. We impute our own mechanics of intelligence to it. It must understand something. It must have built an internal model of its problem domain that works in the way we think. Now that it can do X, it must immediately be able to do Y, because that would be true for pretty much any human.
This imputation is always wrong. Always. And yet we do it every time, without fail. We are hard-wired to make this mistake, just as the computer is hard-wired to be unable to.
Generalising wildly, because I’m a human and that’s what we do, all AI/ML systems are, in the jargon, overfitted. They are inescapably mired in specificity. Some are simple and specific, some — e.g. anything with the adjective ‘Deep’ attached — are hugely complex and specific, but basically all of them will fail given some — to the human observer imperceptible — change in the problem. With current systems for things like image classification,4 the boundaries of what works and what doesn’t can be bewilderingly, fractally complicated, with such a seemingly vast spread on the works side that it looks a lot like generalisation; and yet, give it a tiny nudge and BAM!
It turns out that this fragility is often vulnerable to exploitation. The burgeoning field of adversarial examples demonstrates many ways in which machine learning models can be pushed into getting things wrong, with potentially dangerous consequences.5 If there’s one solid lesson to be learned from the history of computers, it’s that if there’s some way to abuse a system for profit, or even just to be a twat, people will do it. So you can be sure there are plenty of Black Mirror storylines looming in the near future of all this. But those stories will continue to be about what they’ve always been about. Profiteers. Twats. People. And the problem will continue to be what it’s always been. Not artificial intelligence, just natural stupidity.
Anyway, cancel that rope order and cheer yourself up with Gary Marcus undercutting some of the Deep Learning hype. It’s the latest thing, apparently.
1. Stross, that is, not Brooker. Though Black Mirror of course feeds into (and on) this discourse too.
2. Clearly, this can be effective — perhaps more so than costlier, more sophisticated approaches. But it’s not exactly rocket science, is it? At best it’s on the level of “Would you like fries with that?”
3. See, eg, Hofstadter’s 1995 collection on Fluid Concepts and Creative Analogies.
4. This is my actual day job, btw. Though it’s not entirely clear for how much longer.
5. Fun recent examples include road sign bamboozling, a turtle that pretends to be a rifle and stickers that turn anything into a toaster.